Machine Learning Regression Trend
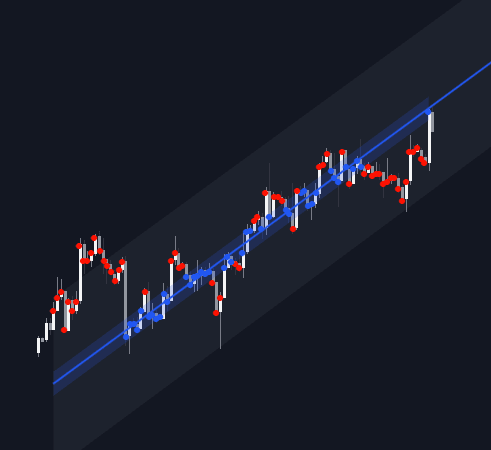
Works on the Following Platforms
The Machine Learning Regression Trend tool uses random sample consensus (RANSAC) to fit and extrapolate a linear model by discarding potential outliers, resulting in a more robust fit.
USAGE
The proposed tool can be used like a regular linear regression, providing support/resistance as well as forecasting an estimated underlying trend.
Using RANSAC allows filtering out outliers from the input data of our final fit, by outliers we are referring to values deviating from the underlying trend whose influence on a fitted model is undesired. For financial prices and under the assumptions of segmented linear trends, these outliers can be caused by volatile moves and/or periodic variations within an underlying trend.
Adjusting the "Allowed Error" numerical setting will determine how sensitive the model is to outliers, with higher values returning a more sensitive model. The blue margin displayed shows the allowed error area.
The number of outliers in the calculation window (represented by red dots) can also be indicative of the amount of noise added to an underlying linear trend in the price, with more outliers suggesting more noise.
Compared to a regular linear regression which does not discriminate against any point in the calculation window, we see that the model using RANSAC is more conservative, giving more importance to detecting a higher number of inliners.
DETAILS
RANSAC is a general approach to fitting more robust models in the presence of outliers in a dataset and as such does not limit itself to a linear regression model.
This iterative approach can be summarized as follow for the case of our script:
- Step 1: Obtain a subset of our dataset by randomly selecting 2 unique samples
- Step 2: Fit a linear regression to our subset
- Step 3: Get the error between the value within our dataset and the fitted model at time t, if the absolute error is lower than our tolerance threshold then that value is an inlier
- Step 4: If the amount of detected inliers is greater than a user-set amount save the model
- Repeat steps 1 to 4 until the set number of iterations is reached and use the model that maximizes the number of inliers
SETTINGS
- Length: Calculation window of the linear regression.
- Width: Linear regression channel width.
- Source: Input data for the linear regression calculation.
RANSAC
- Minimum Inliers: Minimum number of inliers required to return an appropriate model.
- Allowed Error: Determine the tolerance threshold used to detect potential inliers. "Auto" will automatically determine the tolerance threshold and will allow the user to multiply it through the numerical input setting at the side. "Fixed" will use the user-set value as the tolerance threshold.
- Maximum Iterations Steps: Maximum number of allowed iterations.
Trading is risky and many will lose money in connection with trading activities. All content on this site is not intended to, and should not be, construed as financial advice. Decisions to buy, sell, hold or trade in securities, commodities and other markets involve risk and are best made based on the advice of qualified financial professionals. Past performance does not guarantee future results.
Hypothetical or Simulated performance results have certain limitations. Unlike an actual performance record, simulated results do not represent actual trading. Also, since the trades have not been executed, the results may have under-or-over compensated for the impact, if any, of certain market factors, including, but not limited to, lack of liquidity. Simulated trading programs in general are designed with the benefit of hindsight, and are based on historical information. No representation is being made that any account will or is likely to achieve profit or losses similar to those shown.
Testimonials appearing on this website may not be representative of other clients or customers and is not a guarantee of future performance or success.
As a provider of technical analysis tools for charting platforms, we do not have access to the personal trading accounts or brokerage statements of our customers. As a result, we have no reason to believe our customers perform better or worse than traders as a whole based on any content or tool we provide.
Charts used on this site are by TradingView in which the majority of our tools are built on. TradingView® is a registered trademark of TradingView, Inc. www.TradingView.com. TradingView® has no affiliation with the owner, developer, or provider of the Services described herein.
This does not represent our full Disclaimer. Please read our full disclaimer.
© 2025 LuxAlgo Global, LLC.